By Vall Herard, CEO and Co-Founder, Saifr
Artificial intelligence (AI) has rarely been out of the news this century, but it has often been associated with fears about job losses, the growing power of intelligent machines and various apocalyptic scenarios. Decades of science fiction have taught people to be skeptical.
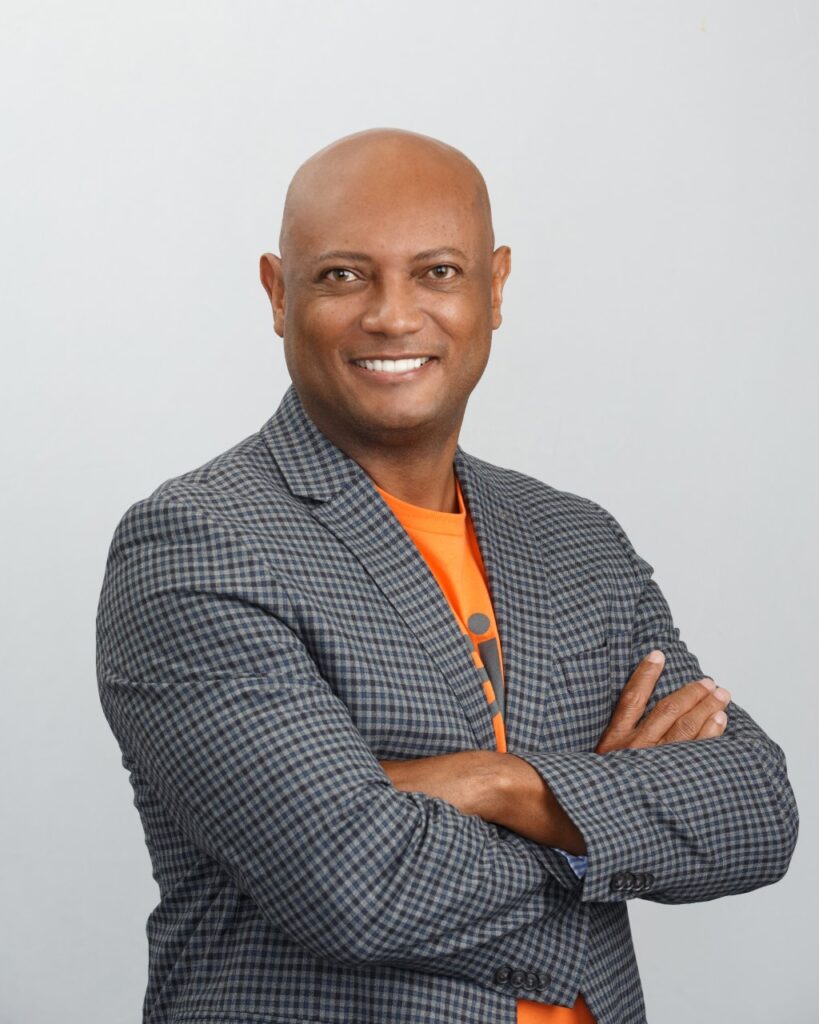
However, the rise of online tools like Open AI’s ChatGPT and image-generating systems like Stable Diffusion, Midjourney, and others, have presented AI in a different light to the once-fearful public. These products are seen as fun, as playthings and inspiration generators, miraculous tools that mimic the work of poets, artists, scientists, and philosophers.
Put another way, some AI providers’ natural-language-based output has begun approaching what a human might produce. These technologies seem not only advanced, but also erudite and wise, because they use the written word – and sometimes use it well.
As a result, the public’s new sense of wonder has quickly become trust, and even laziness. A recent news report suggested that up to 20% of assignments at one university had revealed detectable AI assistance.
In a sense, both outlooks—fear and lazy acceptance—are a problem. This is because they presume that AI is, as the term suggests, a form of general intelligence, even sentience. At present, that is far from the case: today’s machines cannot think, and are not self-aware.
Natural language tools like ChatGPT merely simulate intelligence. They synthesize new content by analyzing a vast amount of existing data and identifying patterns within it. Importantly, that source data has been created by humans, and is now being used to train AI tools (which are themselves programmed by people).
In other words, human logic, creativity, design, and innovation are always front and center of so-called ‘artificial’ tools. And that means human failings, biases, and illogic may be too. So, we must be careful and responsible.
For example, historic data may contain systemic or personal biases that a poorly designed tool may simply reproduce and automate. This is certainly an area that the regulators worry about, and the U.S. government has published its Blueprint for an AI Bill of Rights to protect citizens from harm.
But at a more fundamental level, human logic is not easy to replicate.
Other recent innovations, such as Meta’s Galactica large language model, have revealed another problem: AI sometimes gets things wrong, or exhibits a poor ‘understanding’ of fundamental principles—criticisms that apply to ChatGPT as well.
But remember, these products cannot think, which means they have no comprehension of any of the concepts they seem so adept at communicating.
In Galactica’s case, the AI began inventing non-existent research and attributing it to recognized authors. It was rapidly taken offline.
For organizations that are exploring the technology’s genuine potential to be transformative, to unlock insights and patterns from data—and help solve real, serious problems—faddy apps and a distracted public are unhelpful.
What’s needed is consistent and thoughtful human design, feedback and interaction so we can build AI that is viable, useful and reliable in the long term. And importantly, that can work alongside humans rather than sweep them aside.
Countless tasks require human judgement, empathy, expertise, experience, decision-making and skill. Such qualities cannot simply be outsourced to an app!
In an ideal world, AI should eliminate unnecessary tasks, not replace human insight. And it should complement or augment what we do best, such as by looking for patterns in big data that might take decades—even centuries—of human analysis to find.
As for AI itself, our industry needs to be more responsible. A human touch is required to determine what data goes into these algorithms.
The huge volumes of data needed to train an AI require intensive management. Moreover, that data needs to be massaged by humans to eliminate conscious or unconscious bias, and sometimes the all-too-human mistakes of the past.
The challenge is that biases can be subtle: some analysts may miss them or introduce new ones of their own. Arguably, to be human is to be biased, because everyone has a point of view. But AI needs to work for everyone, regardless of that.
This human touch, testing with human help and comparison with human processes, is a critical part of the investment that makes an AI product viable.
This is why a ‘human parallel’ test is advisable: give human experts the same set of problems as the AI, then compare the results and move forward incrementally.
It is essential, too, to maintain constant vigilance and regular updates: a slower and more expensive option, perhaps, but I believe always the right one. A policy which acknowledges that, without a significant investment of human activity up front, AI models will not be strong enough to survive.
So, the key lesson is this: AI needs human validation and support, but it should never make humans invalid.
The opinions provided are those of the author and not necessarily those of Fidelity Investments or its affiliates. Fidelity does not assume any duty to update any of the information. Fidelity and any other third parties are independent entities and not affiliated. Mentioning them does not suggest a recommendation or endorsement by Fidelity.